DATA SCIENCE
Delivering information is not just about orderly distribution for the masses. Organizations need to maintain their competitive edge by making new discoveries. Data Science is the discipline of extracting knowledge from the data landscape. This realm of discovery has a completely different set of requirements from the standard data-to-information life-cycle. What this often means is confusion on how to work with data. Intricity brings a best of breed framework for building harmony between Data Science and Business Intelligence. This at times requires both a cultural shift as well as a better understanding of the interplay of how the data landscape comes together.
The Data Science and Machine Learning teams not only need unstructured access to data, they also need the ability to experiment with external data sets. Unlike aggregate analytics, often Data Science teams are attempting to model reality by iterating through raw situations, the more successful these teams are, the better they are at predicting the future and optimizing inputs. Intricity's Data Moat Strategy is a springboard to help the organization develop a data landscape that produces a defensible position in the machine learning marketplace, and makes the most of the organizations data assets.
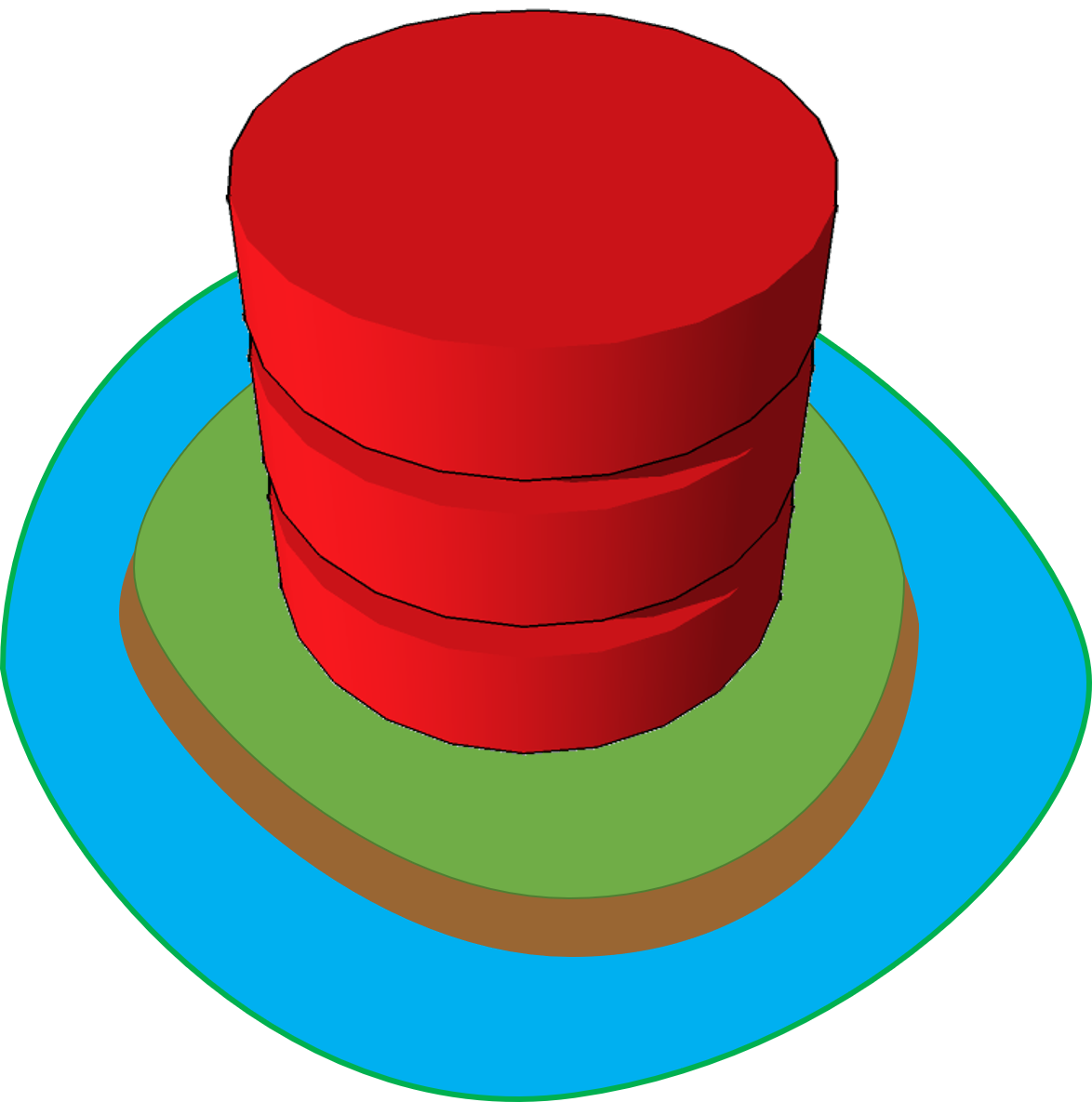
TO BUSINESS PROFESSIONALS
Data Scientists can't do much with generic descriptions of success. They need to be able to train models based on organizational goals that are well articulated, and have identifiable positive and negative indicators in the data. Additionally, they need to be able to have simplified access to data assets. Intricity's Data Science team can help you build a Data Moat which will defend your organizations business interests by ensuring you are staying ahead of market discoveries. A Data Moat is a strategic data set specifically assembled to enable to extract knowledge via statistics and machine learning. Obtaining such a data set is increasingly becoming the linchpin to obtaining a defensible position against hungry competitors.
TO TECHNICAL PROFESSIONALS
The architecture for a data science team is not segregated to just statistical tools and raw compute. The architecture needs to contain a component that gracefully deploys new knowledge to the rest of the organization. Your organizations data lake and data warehouse should work hand in hand to that end. If your data science team is wasting time integrating the same data to do the same analytics than you're falling into a scalability trap. Yes your playground SHOULD be the data lake, but that does not mean that you don't pass on your discoveries to the data warehouse, so you keep yourself out of the integration scalability trap.
Intricity can help you scale your Data Science discoveries so that you keep your head in the innovations rather than the mundane integration tasks. Additionally, Intricity can help you build a bridge with your business stakeholders to ensure their requirements map to more effective machine learning models that don't require excessive pruning, and thus require less processing cycles.
TALK WITH A SPECIALIST