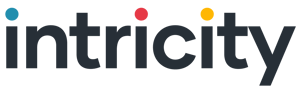
Case Study: Transforming Water with Snowflake's Data Platform
Written by Jared Hillam
About Customer
[The Client] is an innovative North American water treatment organization with well over 15,000 industrial and municipal sites. Leveraging its leading solutions in water management and recycling, [The Client] has become a leader which local municipalities look to. With its growing portfolio of water management assets, [The Client] has emerged as one of the foremost authorities in water management and environmental protection.
Challenges
The North American water landscape is rife with consolidation opportunities. Currently over 15,000 small water municipalities exist. The aging water infrastructure in North America and the drive for increased water quality presents a consolidation opportunity to the organizations most prepared to manage it. [The Client] is preparing every facet of its enterprise to meet that challenge, both with its existing and future facilities.
As clean water sources become less scarce and as aging infrastructures begin to leak, having an end-to-end understanding of the health and integrity of a municipalities water network is paramount. [The Client] was faced with attempting to answer inherently scientific questions about its industry without having a consolidated perspective of their water treatment and distribution networks. The amount of data coming from the industrial and municipal sites made for a daunting integration task. The need to conform this data onto a scalable data platform was evident.
One of the high priority analytics [The Client] needed was to determine where the shrinkage was in their water networks. This shrinkage at times happens through natural evaporation, but it also had many other causes like leaks and sometimes bypassed water meters. Prior attempts to determine non-billed water were done through high-level estimations on a monthly level. To address the non-revenue water, a much deeper and more integrated data analysis would be required to get tighter timeframes and geographies.
Navigating Constraints
- High number of source systems feeding data
- Building a culture around cloud adoption for a data platform
- Data feeds of meters were often at a very high grain
- Shifting the organization and even the industry to think in terms of hours instead of months
Win 1: Robust Scalability
[The Client] and Intricity architected a future state architecture that could scale well beyond the confines of its current book of business. This robust scalability made it possible to offer its data solutions as a platform to its municipality clients so [The Client] could track water usage at a much deeper level of detail.
The unlimited capacity of data storage and compute in Snowflake provided a solid backbone to deal with whatever new sources of data would come their way. The simplicity of FiveTran for data replication made the variety of sourcing data much easier to deal with, and the agility of the BladeBridge Studio code production made the standing up of the integration logic much faster to build. This combination of technologies provided a scalable and portable solution.
Win 2: Water Tracking at an Hourly Level
[The Client] and Intricity worked together to redefine the measurement standards for the entire industry. Time lapses traditionally were tracked on a monthly level, and in many cases the devices themselves didn't have grains of data lower than this measure. There are physical reasons that this standard existed. Water meters around North America traditionally provided meter (AMR) data via a long range RFID which get triggered by utility trucks configured to collect that data. On a monthly basis, the meter trucks would drive their routes to collect data for billing purposes. About a decade ago, a new architecture for data collection was introduced called Automated Metering Infrastructure (AMI). This architecture provided access to hourly meter feeds, and across North America there is a mix of AMR, AMI and even physical meter reads in the infrastructure.
Through the development of this consolidated data architecture, [The Client] and Intricity came up with a highly accurate way to allocate hourly data patterns to the AMR and physical read meters using patterns and algorithms derived from the AMI data.
Win 3: Hourly Non-Revenue Water
One of the low hanging fruits for the consolidation of data was the tracking of Non-Revenue Water. This category of water represents water that is not billed to the network because it is lost to a number of factors including natural evaporation, leaks in the network, or theft via bypassed meters. With the consolidated data platform, [The Client] and Intricity came up with a wide variety of forensic analytics models that could determine where the water was, why it was lost, and where it was lost.
Win 4: Exacting Costs of Water in the Network
Most water municipalities don’t have data at a level sufficient to attribute the actual cost of every gallon of water in the network. Therefore, most organizations treat the cost of a gallon the same as any other. However, depending on how the water is produced, the value of each gallon can vary quite differently. For example, water coming from a reservoir has a different cost to produce compared to a gallon pumped out of the ground. Analyzing the production costs and aligning those costs to the meters where the water is produced allowed [The Client] the ability to know the cost of every gallon in the network. This gallon traceability and detailed cost allocation provides an enhanced level of visibility and business priority for decision and business process optimization. As such it is rewriting the books in the water industry for measuring storage and distribution.
Win 5: Data Enrichment with Weather Data
By introducing weather data, [The Client] and Intricity were able to incorporate new analytics which provided excellent context for Non-Revenue Water at the consumer level. On hot/dry periods, customers typically increase their consumption to water their lawns. In cases where that does not occur it could be for a number of reasons. The customer could be letting their lawn die in the sun, in which case their lawn would be brown. In cases where meter consumption does not rise, there are other factors that may be in play. Either there is a local leak in the water main, or the home may have bypassed the meter when the sprinkling system was installed. By leveraging the weather data as event markers, [The Client] was able to identify potential Non-Revenue water targets.
Win 6: Notification of Potential Leaks
By being able to trace trends in consumption, [The Client] and Intricity came up with an ability to flag irregularities in metered consumption patterns, which could be indicators of leaks at a customers home. This provides its municipal customers the ability to conserve water, and be a good steward over the customers spend.
Who is Intricity?
Intricity is a specialized selection of over 100 Data Management Professionals, with offices located across the USA and Headquarters in New York City. Our team of experts has implemented in a variety of Industries including, Healthcare, Insurance, Manufacturing, Financial Services, Media, Pharmaceutical, Retail, and others. Intricity is uniquely positioned as a partner to the business that deeply understands what makes the data tick. This joint knowledge and acumen has positioned Intricity to beat out its Big 4 competitors time and time again. Intricity’s area of expertise spans the entirety of the information lifecycle. This means when you’re problem involves data; Intricity will be a trusted partner. Intricity's services cover a broad range of data-to-information engineering needs:
What Makes Intricity Different?
While Intricity conducts highly intricate and complex data management projects, Intricity is first a foremost a Business User Centric consulting company. Our internal slogan is to Simplify Complexity. This means that we take complex data management challenges and not only make them understandable to the business but also make them easier to operate. Intricity does this through using tools and techniques that are familiar to business people but adapted for IT content.
Thought Leadership
Intricity authors a highly sought after Data Management Video Series targeted towards Business Stakeholders at https://www.intricity.com/videos. These videos are used in universities across the world. Here is a small set of universities leveraging Intricity’s videos as a teaching tool:
Talk With a Specialist
If you would like to talk with an Intricity Specialist about your particular scenario, don’t hesitate to reach out to us. You can write us an email: specialist@intricity.com
(C) 2023 by Intricity, LLC
This content is the sole property of Intricity LLC. No reproduction can be made without Intricity's explicit consent.
Intricity, LLC. 244 Fifth Avenue Suite 2026 New York, NY 10001
Phone: 212.461.1100 • Fax: 212.461.1110 • Website: www.intricity.com
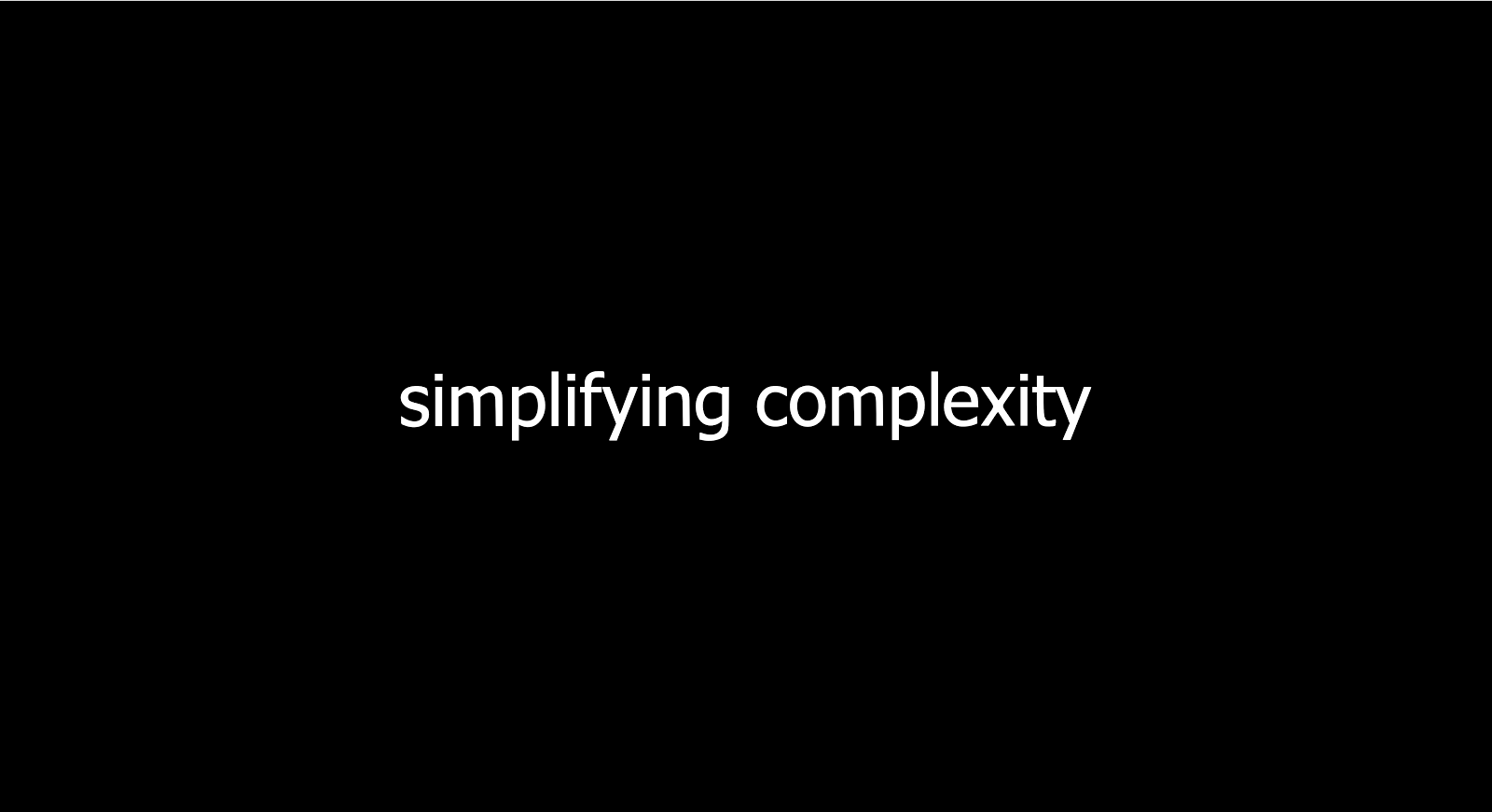