About Client
The Client is a national weight-loss brand that specializes in delivering healthy foods to its subscribers. They have helped millions of people in North America obtain their weight loss goals and have become a household name brand.
Challenge
The extensive data gathering and analytics requirements produced by their marketing campaigns were growing the Client's data footprint and its associated compute in a linear path with extreme jumps. This linear growth exploded with the deployment of a marketing application that simply did not have the appropriate optimization and spiked their Snowflake compute and storage out of control. The cost of their compute expansion caught the attention of their executive stakeholders as it was trending to millions of dollars in cost overruns. The attention from the executive stakeholders drove the organization to seek expertise in Snowflake optimization to cut off the linear growth. This was the status the client was in when Intricity started its optimization work.
Navigating Constraints
- The average critical query was consuming between 12,000 and 40,000 micro-partitions. Additionally, these queries were running all day long with updated data.
- The churn of data was very high due to its real-time nature with some tables churning several thousand times per day.
- The Client's time travel data retention was very high and added complexity to the price reduction of the Snowflake footprint.
- The marketing tool validates all of the query structure every time it is queried which is a cost that cannot be circumvented.
Win 1 :
Intricity immediately began a cleanup of known performance problems with some tactical efforts around the existing warehouses. The team needed to provide a ceiling and floor range that could guide the finance teams in determining the compute commit that should be made for the next contract. The tactical changes gave some confidence that the range was not jumping on a linear path while still satisfying the needs of the user community.
Win 2:
Upon stabling the compute footprint, Intricity began a strategic table optimization track that could apply automation to efficiently pack the Client's tables for the fastest possible acquisition speeds and lowest amount of micro-partition scanning. This packing was based on the most optimal clustering sequence for the highest possible performance. Intricity leveraged its FlowObjects tooling to cyclically analyze and repack tables ensuring both the range and the trend stabilized below the negotiated amount.
Win 3:
Intricity generated a data onboarding strategy coupled with automated scripts to deal with blind updates which were sharding the micro-partitions in Snowflake. This leveraged the best practices of the Landing Zone and graduated data to ensure effective packing of the micro-partitions after the updates had been reconciled. In combination with the clustering, the queries themselves were optimized to take better advantage of the structural changes. In many cases, the query speeds went from minutes to seconds.
Win 4:
Intricity implemented the FlowObjects tracking framework which indicates where tables are beginning to get out of control and suggests changes. The cost control provided by this framework has enabled the client to stay well below the contracted amount (refer back to the yellow line).
This additional capacity has given the organization the ability to trial new content with a process that accounts for future growth.
Who is Intricity?
Intricity is a specialized selection of over 100 Data Management Professionals, with offices located across the USA and Headquarters in New York City. Our team of experts has implemented in a variety of Industries including, Healthcare, Insurance, Manufacturing, Financial Services, Media, Pharmaceutical, Retail, and others. Intricity is uniquely positioned as a partner to the business that deeply understands what makes the data tick. This joint knowledge and acumen has positioned Intricity to beat out its Big 4 competitors time and time again. Intricity’s area of expertise spans the entirety of the information lifecycle. This means when you’re problem involves data; Intricity will be a trusted partner. Intricity's services cover a broad range of data-to-information engineering needs:
What Makes Intricity Different?
While Intricity conducts highly intricate and complex data management projects, Intricity is first a foremost a Business User Centric consulting company. Our internal slogan is to Simplify Complexity. This means that we take complex data management challenges and not only make them understandable to the business but also make them easier to operate. Intricity does this through using tools and techniques that are familiar to business people but adapted for IT content.
Thought Leadership
Intricity authors a highly sought after Data Management Video Series targeted towards Business Stakeholders at https://www.intricity.com/videos. These videos are used in universities across the world. Here is a small set of universities leveraging Intricity’s videos as a teaching tool:
Talk With a Specialist
If you would like to talk with an Intricity Specialist about your particular scenario, don’t hesitate to reach out to us. You can write us an email: specialist@intricity.com
(C) 2023 by Intricity, LLC
This content is the sole property of Intricity LLC. No reproduction can be made without Intricity's explicit consent.
Intricity, LLC. 244 Fifth Avenue Suite 2026 New York, NY 10001
Phone: 212.461.1100 • Fax: 212.461.1110 • Website: www.intricity.com
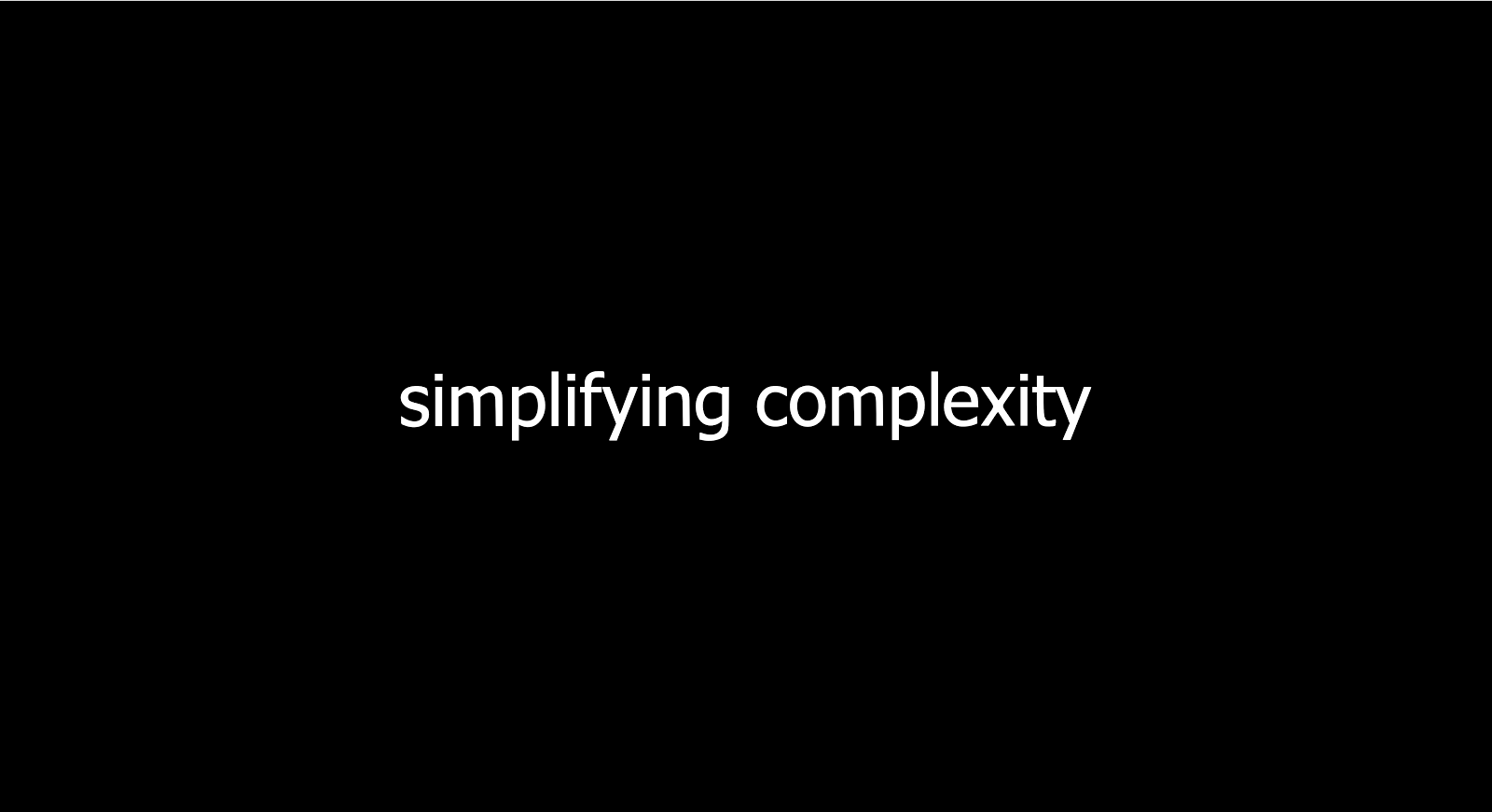