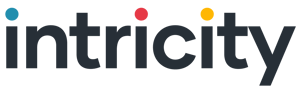
Case Study: 10X Improvement in Snowflake Performance
Written by Jared Hillam
About The Client
The Client today operates some of the largest web properties and brands in the world. Their portfolio includes household names for news, video, investment information, and home services sites as well as a host of other brands. Additionally, the Client is a major share holder in many other web properties, which it actively invests in.
Challenges
The Client decided to attempt their Snowflake deployment independently. While there was an excellent team, the size of the data footprint surfaced challenges to the deployment that they internally assumed were issues with Snowflake. Snowflake made an introduction to the Intricity team to change the trajectory of their compute usage and to fix the incorrect perceptions.
The challenges included inefficiencies in automating the data replication process, sub-optimal data modeling practices, and disorderly approaches to their data load leading to excessive micro-partition scans. These issues normally wouldn't manifest as a road blocker in Snowflake for most clients, but the Client’s footprint of data was so tremendous that the only way they could get performance was a massive allocation of warehouse compute resources to produce queries quickly. This allocation was well above the forecasted spend.
Navigating Constraints
- Forecasted budget strains: At the rate of credit consumption, the Client was entertaining the idea of de-scoping the project. As part of onboarding with Intricity, the Client set a "compute goalpost" as a decision point.
- Very large quantities of data: With a leading portfolio of web brands, the Client's properties not only contained very high traffic rates, but they also captured every little event during each browsing session, which made very high volume data feeds.
- Skeptical technical teams: While the teams were very capable, the "DIY" approach had not been done with a deep experience in Snowflake best practices, but were rather more oriented to HDFS techniques.
Win 1: Increasing query speed & Efficiency by over 10X
Intricity conducted an analysis on the Client’s consumption patterns to determine the usage of some of their largest tables. The date column was one of the largest which was attached to the most queries. Using this information, Intricity and the Client redesigned the loading orchestration to sort the data by the date dimension for their loads, at the time Auto Clustering was not available, so the sorting of the data was done as a reload of the tables.
While this change required some rework, it increased the overall efficiency and performance of Snowflake by over 10X.
Win 2: Snowpipes for Near Real-Time Onboarding of Data
The volumes of data being onboarded from their websites was a constant stream and came in large tranches. Intricity and the Client worked together to deploy Snowpipes to automate the onboarding process and do so in a triggered manner rather than in massive batches. By coupling this with their integration processes, the Client was able to orchestrate a near real-time dimensionalized load of data.
Win 3: Education on Modeling and Snowflake Operation
The Client’s very capable team was soaking up the information like a sponge during the execution of the project. The Intricity Solution Architects outlined modifications in the Client’s data model which also greatly improved the query performance as well as end-user access to the data.
Additionally, the Client retained the Intricity Solution Architects as a sounding board to validate how to maintain optimization of the Snowflake landscape.
The Client was not only able to be well under their credit consumption "goal post" but also the team was able to sustain the performance independently.
Who is Intricity?
Intricity is a specialized selection of over 100 Data Management Professionals, with offices located across the USA and Headquarters in New York City. Our team of experts has implemented in a variety of Industries including, Healthcare, Insurance, Manufacturing, Financial Services, Media, Pharmaceutical, Retail, and others. Intricity is uniquely positioned as a partner to the business that deeply understands what makes the data tick. This joint knowledge and acumen has positioned Intricity to beat out its Big 4 competitors time and time again. Intricity’s area of expertise spans the entirety of the information lifecycle. This means when you’re problem involves data; Intricity will be a trusted partner. Intricity's services cover a broad range of data-to-information engineering needs:
What Makes Intricity Different?
While Intricity conducts highly intricate and complex data management projects, Intricity is first a foremost a Business User Centric consulting company. Our internal slogan is to Simplify Complexity. This means that we take complex data management challenges and not only make them understandable to the business but also make them easier to operate. Intricity does this through using tools and techniques that are familiar to business people but adapted for IT content.
Thought Leadership
Intricity authors a highly sought after Data Management Video Series targeted towards Business Stakeholders at https://www.intricity.com/videos. These videos are used in universities across the world. Here is a small set of universities leveraging Intricity’s videos as a teaching tool:
Talk With a Specialist
If you would like to talk with an Intricity Specialist about your particular scenario, don’t hesitate to reach out to us. You can write us an email: specialist@intricity.com
(C) 2023 by Intricity, LLC
This content is the sole property of Intricity LLC. No reproduction can be made without Intricity's explicit consent.
Intricity, LLC. 244 Fifth Avenue Suite 2026 New York, NY 10001
Phone: 212.461.1100 • Fax: 212.461.1110 • Website: www.intricity.com
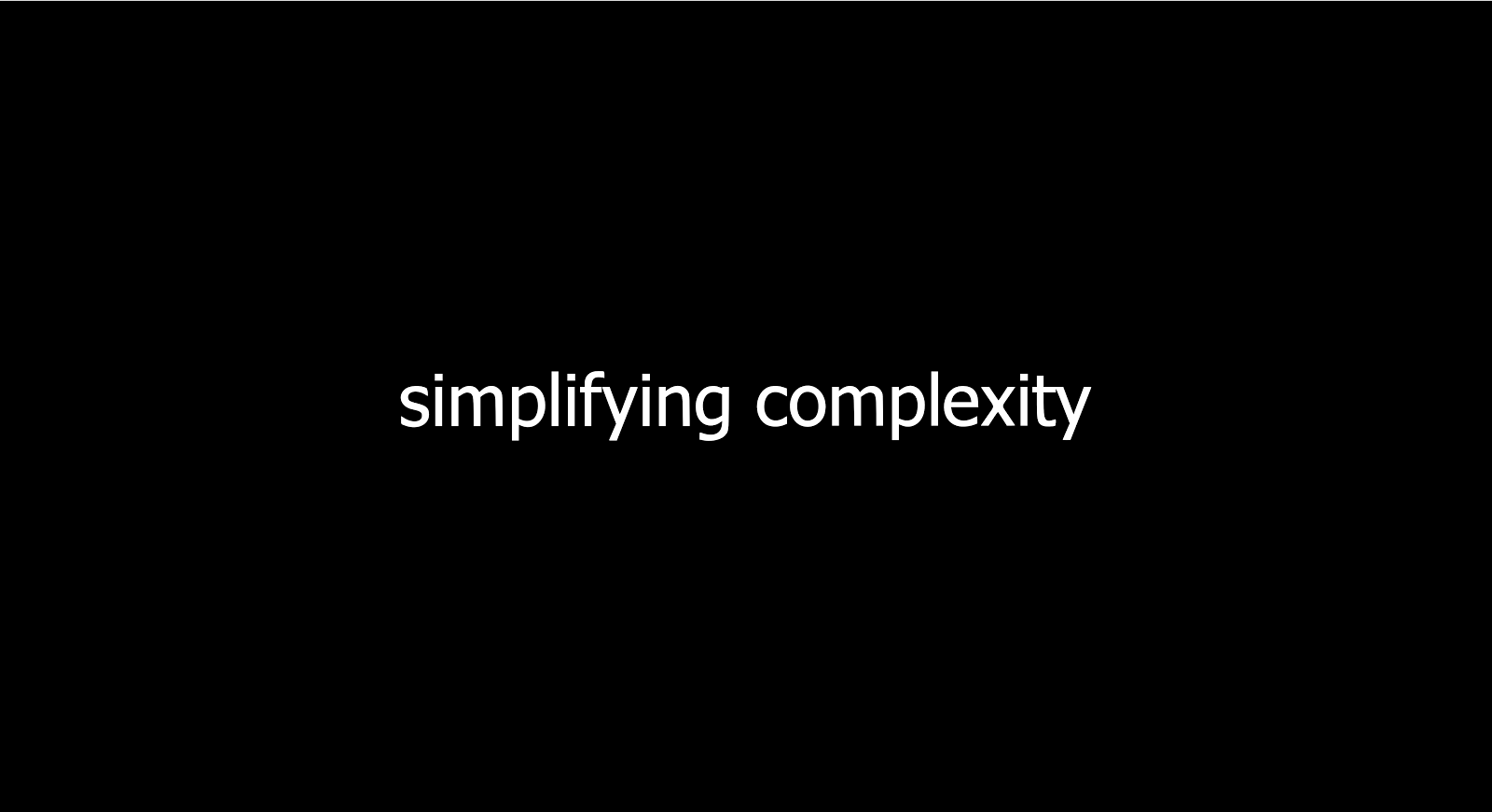